Sent Successfully.
Home / Blog / Artificial Intelligence / BERT vs GPT: Comparison of Two Leading AI Language Models
BERT vs GPT: Comparison of Two Leading AI Language Models
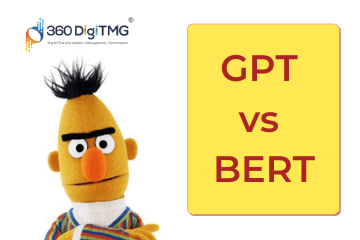
Table of Content
The future and constant discoveries made in Deep Learning are quite exciting. It is very possible soon, machine learning tools capable of producing human language will be used to generate a large amount of content and as a reader, you would never realize whether the content is written by a human or by an AI-generated bot. Such is the power of Natural language processing models. GPT3 is a well-known machine learning tool that is capable of sustaining “freakishly natural conversations” as described by some of the researchers. Consider a situation, where you are enjoying a deep philosophical conversation, only to discover that it was a machine you were communicating with. Or a customer service agent providing a natural, high quality, and intuitive interaction with customers and even solving their queries with a range of responses only to find out that is a chatbot. The use cases are countless where natural language models can be applied. Click here to learn Data Science in Hyderabad
Their impact can provide an almost human touch to any kind of interaction. Along with GPT (Generative Pre-trained Transformer), BERT (Bidirectional Encoder Representations from Transformers) is credited as one of the earliest pre-trained algorithms to perform Natural Language Processing (NLP) tasks. Since then research in this area is advancing and we have seen increased progress in technology. Most of the NLP models belong to a Transformer architecture family that uses ‘Attention Mechanism’ techniques. ‘Attention’ became a significant element in language models. It also became the basis of GPT. GPT Transformers are language models known to produce text like humans. Both BERT and GPT became the most popular deep learning model achieving state-of-the-art across many NLP tasks. Click here to learn Data Science in Bangalore
While Transformers, in general, have reduced the amount of data required to train NLP models, GPT has a distinct advantage over BERT as it requires very few examples of data to train the model. Both pre-trained NLP models share many similarities, this article will understand an overview of each model, along with its comparison.
BERT was developed by Google in 2018, after its release, BERT’s capability achieved a General Language Understanding Evaluation (GLUE) score of 93% accuracy on Stanford competitive QA dataset. (SQuAD). After the launch of GPT-3 by OpenAI, it surpassed the capabilities and accuracy of its previous versions and NLP models. It is considered the next wave of AI. Similar to BERT, GPT-3 is also a large transformer-based architecture trained on billions of parameters. Its capabilities have showcased extraordinary performances for several NLP tasks such as language translation, unscrambling words, Q&A, including generating content, codes, solving arithmetic problems, compose song lyrics, write a movie script, stories, poems, and much more. The purpose of GPT-3 was to reduce the complexity of machine learning models to accomplish simple natural language tasks. GPT was capable of solving tasks that were new and not encountered before as long as it is pre-trained on large data making it one of the potential tools that can revolutionize machine learning across the NLP landscape. Click here to learn Data Analytics in Bangalore
Learn the core concepts of Data Science Course video on YouTube:
The figure below further compares GPT-3 and BERT based on three dimensions Architecture, size, and learning approach:
Figure 1 How is GPT-3 different from BERT (Source: evdelo, Future of AI text generation)
Bidirectional BERT and Autoregressive GPT
Trained on billions of words BERTs main advantage is that it utilizes bi-directional learning to gain context of words, meaning it understands the context of words by reading it both ways from left to right and right to left simultaneously. It is trained on long dependencies between the text of various contexts. BERT works on encoding mechanisms to generate language. Unlike BERT, GPT models are unidirectional, their advantage is the sheer volume of words it is pre-trained on. This allows users to fine-tune NLP tasks with very few examples to perform a given task. GPT relies on the decoder part of the transformer architecture to generate text. It relies on previous values to predict current values. The bidirectional model on the other hand learns context based on the words around it instead of just relying on the word before or after the considered word. Click here to learn Data Analytics in Hyderabad
Size Comparison
In terms of size GPT-3 is enormous compared to BERT as it is trained on billions of parameters ‘470’ times bigger than the BERT model. BERT requires a fine-tuning process in great detail with large dataset examples to train the algorithm for specific downstream tasks. BERT architecture has ‘340’ million parameters compared to 175 billion parameters of GPT-3. The average user may run out of memory in an attempt to run the GPT model. Its size is breath-taking, which makes it powerful in solving language tasks with realistic results, it can create fiction, even develop programmers' code, generate thoughtful articles, summarize text and perform a wide range of tasks.
Fine Tuning Capability
Fine-tuning models require enormous amounts of data and also needs tuning and updating model weights to accurately solve problems. BERT requires fine-tuning and can be leveraged to perform specific tasks. GPT-3 from OpenAI on the other hand is where pre-trained models can be used to solve downstream tasks without modifying the architecture. GPT-3 has been trained on a large diverse dataset containing billions of texts. The model generates meaningful paragraphs of text and has achieved competitive state-of-the-art results on a wide variety of tasks. It adopts a unique learning approach where there is not much need for labeled data. Instead, GPT-3 is capable of learning from no data (also known as zero-shot learning), using one example data (one-shot learning) or few example data (few-shot learning). Fine-tuning is a complex process by eliminating the steps of fine-tuning. The advanced version of GPT(GPT3) holds an attractive prospect compared to BERT, where users can just explain the task once, and with just a click, they can create remarkable applications. Click here to learn Artificial Intelligence in Bangalore
Availability
BERT is an open-source tool and easily available for users to access and fine-tune according to their needs and solve various downstream tasks. GPT-3 on the other hand is not open-sourced. It has limited access to users and it is commercially available through API. A beta version is released to limited users upon request. The Open AI API provides a “Text in, Text Out” interface allowing users to experiment with NLP tasks and even incorporate with other tools to develop new applications and further experiment with the technology. Open AI provides a range of price options aside from a limited trial period to subscribe and gain access to the GPT interface. Click here to learn Artificial Intelligence in Hyderabad
Risks
Machine Learning is moving at a very fast pace of progress, where there are constant upgrades and surprising discoveries both positive and negative. The powerful capabilities of AI can be accessed for harmful-use cases, such as spamming, fake news, transcripts that can sound incredibly convincing, mimic people of power, generating malicious codes or content around sensitive topics that can cause outrage, or panic, and many other unethical use cases. As researchers, we need to anticipate all possible consequences of emerging technology and contribute towards building tools that help better control content and develop safety-relevant activities that can analyze, mitigate and prevent harmful events in the community and focus more on building human-positive AI systems. However impressive these tools may sound; they do make mistakes and have limitations. The AI tools are not known to have a moral stand or obligation and the text produced by NLP models is often so well-written that it becomes easy for people to believe and spread the word. As a result, some restrictions were added to the release of GPT3. Click here to learn Machine Learning in Hyderabad
In conclusion, the benefit of GPT-3 against BERT is that it does not require enormous data for training. As a result, GPT-3 has become a preferred language modeling tool for its advanced capabilities. It provides a different learning approach, where there is no need for large labeled data for solving new tasks. This advancement brought greater potential for organizations, researchers, and users to automate many routine tasks while reducing the speed of processes. While NLP tools are considered state-of-the-art language processing technology there are many opportunities, challenges, and limitations emerging that need to be observed and addressed to achieve remarkable artificial intelligence. Click here to learn Machine Learning in Bangalore
Click here to learn Data Science Course, Data Science Course in Hyderabad, Data Science Course in Bangalore
Data Science Placement Success Story
Artificial Intelligence Training Institutes in Other Locations
Ahmedabad, Bangalore, Chengalpattu, Chennai, Hyderabad, Kothrud, Noida, Pune, Thane, Thiruvananthapuram, Tiruchchirappalli, Yelahanka, Andhra Pradesh, Anna Nagar, Bhilai, Calicut, Chandigarh, Chromepet, Coimbatore, Dilsukhnagar, ECIL, Faridabad, Greater Warangal, Guduvanchery, Guntur, Gurgaon, Guwahati, Indore, Jaipur, Kalaburagi, Kanpur, Kharadi, Kochi, Kolkata, Kompally, Lucknow, Mangalore, Mumbai, Mysore, Nagpur, Nashik, Navi Mumbai, Patna, Porur, Raipur, Salem, Surat, Thoraipakkam, Trichy, Uppal, Vadodara, Varanasi, Vijayawada, Vizag, Tirunelveli, Aurangabad
Navigate to Address
360DigiTMG - Data Science Course, Data Scientist Course Training in Chennai
D.No: C1, No.3, 3rd Floor, State Highway 49A, 330, Rajiv Gandhi Salai, NJK Avenue, Thoraipakkam, Tamil Nadu 600097
1800-212-654-321